
- Our AI Agents
- About us
- Blog
- DeveloperComing soon
- Docs
- Enter MESH App
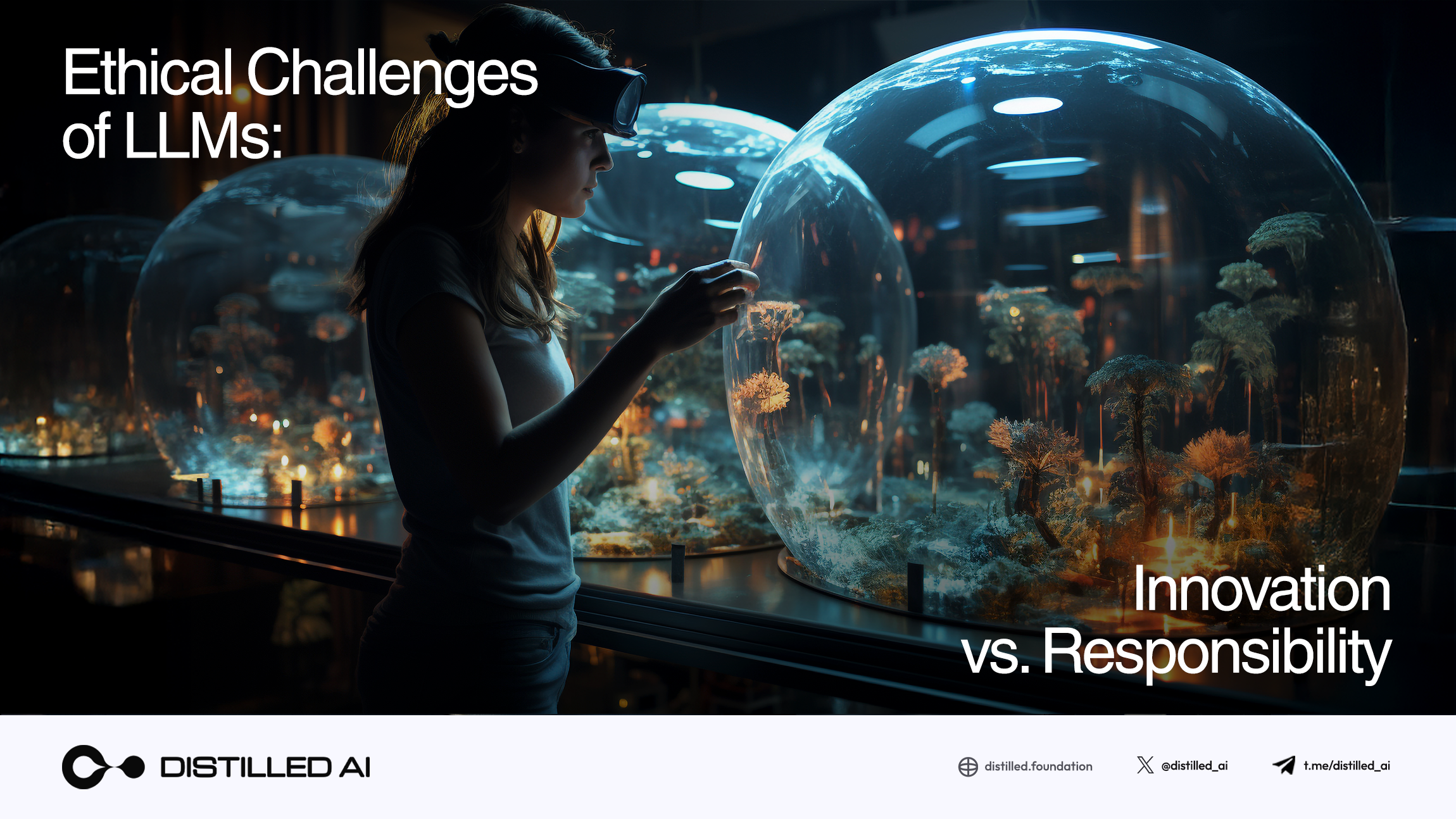
Ethical Challenges of Large Language Models: Balancing Innovation with Responsibility
APR 04, 2025
Large Language Models (LLMs) have propelled AI applications forward—powering advanced chatbots, content generation, and conversational agents. Yet, alongside these leaps in innovation comes a growing concern for ethics and responsibility. From bias to misinformation and user privacy, LLMs can inadvertently raise serious moral and social dilemmas. Below, we look at the key ethical challenges posed by LLMs and discuss strategies to balance cutting-edge development with trustworthiness and accountability.
1. Data Privacy and Consent
Why It Matters
LLMs are trained on enormous datasets, often culled from public online sources like web pages, forums, and social media posts. Personal data can end up in the training corpus, posing privacy threats if names, addresses, or other sensitive details become retrievable by the model.
Mitigation Strategies
• Data Scrubbing: Remove or anonymize personal identifiers during data collection.
• Ethical Data Sourcing: Obtain user consent or rely on robust legal frameworks that allow data usage while respecting individual rights.
• Differential Privacy: Integrate techniques that obscure individual data points to protect personal information.
Outcome: Responsible data practices safeguard user trust and ensure that innovation does not come at the expense of privacy.
2. Bias and Fairness
How Bias Emerges
Training data can reflect societal biases—such as discriminatory language or stereotypes—leading LLMs to perpetuate unfair or harmful outputs. This can manifest as gender, racial, or cultural biases, undermining AI’s objectivity and inclusiveness.
Minimizing Bias
• Diverse Datasets: Curate more balanced, culturally diverse training data.
• Model Audits: Continuously test LLM responses for bias and incorporate corrective measures.
• Explainable AI: Provide transparency into how models arrive at specific outputs, enabling more informed oversight.
Significance: By confronting bias head-on, developers ensure LLMs uphold fairness and equity across all user interactions.
3. Misinformation and Disinformation
The Challenge
Because LLMs generate text by predicting plausible words rather than verifying facts, they can inadvertently produce misleading or outright false information. In high-stakes domains—like health advice, financial guidance, or political discourse—this can be dangerous.
Countermeasures
• Human-in-the-Loop: Employ expert reviewers or moderators for crucial content areas.
• Real-Time Fact-Checking: Integrate external validation systems to identify potential inaccuracies.
• Model Fine-Tuning: Continuously refine the model’s knowledge of verified sources.
Impact: Taking proactive steps against misinformation preserves the integrity of AI outputs and reduces harmful societal effects.
4. Accountability and Governance
Why Governance is Essential
When an LLM’s output causes harm, accountability can be murky—who bears responsibility for the consequences: developers, data providers, or users? Clear governance frameworks help delineate roles and liabilities.
Best Practices
• Policy Transparency: Outline usage guidelines and disclaimers clarifying the intended scope and limitations of LLM-based services.
• Regulatory Compliance: Track emerging regulations (e.g., proposed EU AI Act) and adapt LLM deployments for ongoing legal conformity.
• Ethical Review Boards: Assemble interdisciplinary teams to evaluate policy decisions, model deployments, and incident responses.
Takeaway: Establishing clear lines of responsibility fosters a trustworthy environment, benefiting all stakeholders—developers, users, and society at large.
Large Language Models epitomize the potential of AI-driven innovation, yet come with ethical challenges that demand attention. By prioritizing data privacy, mitigating bias, countering misinformation, and embedding robust governance, organizations can wield LLMs to their fullest potential without undermining public trust. Achieving a balance between state-of-the-art AI advancement and responsible development will be key to ensuring LLMs positively shape the future of communication, education, and societal progress.
Key Takeaways
1. Privacy Matters: Employ data-scrubbing and consent-driven approaches to protect personal information.
2. Confronting Bias: Curate diverse datasets and regularly audit model outputs for discriminatory patterns.
3. Preventing Misinformation: Combine fact-checking tools with careful human oversight in critical contexts.
4. Establishing Accountability: Transparent policies and governance structures clarify responsibilities and uphold trust.
By embracing responsible strategies in LLM development, AI builders not only uphold ethical standards but also strengthen the foundation for sustainable, user-centric innovation.
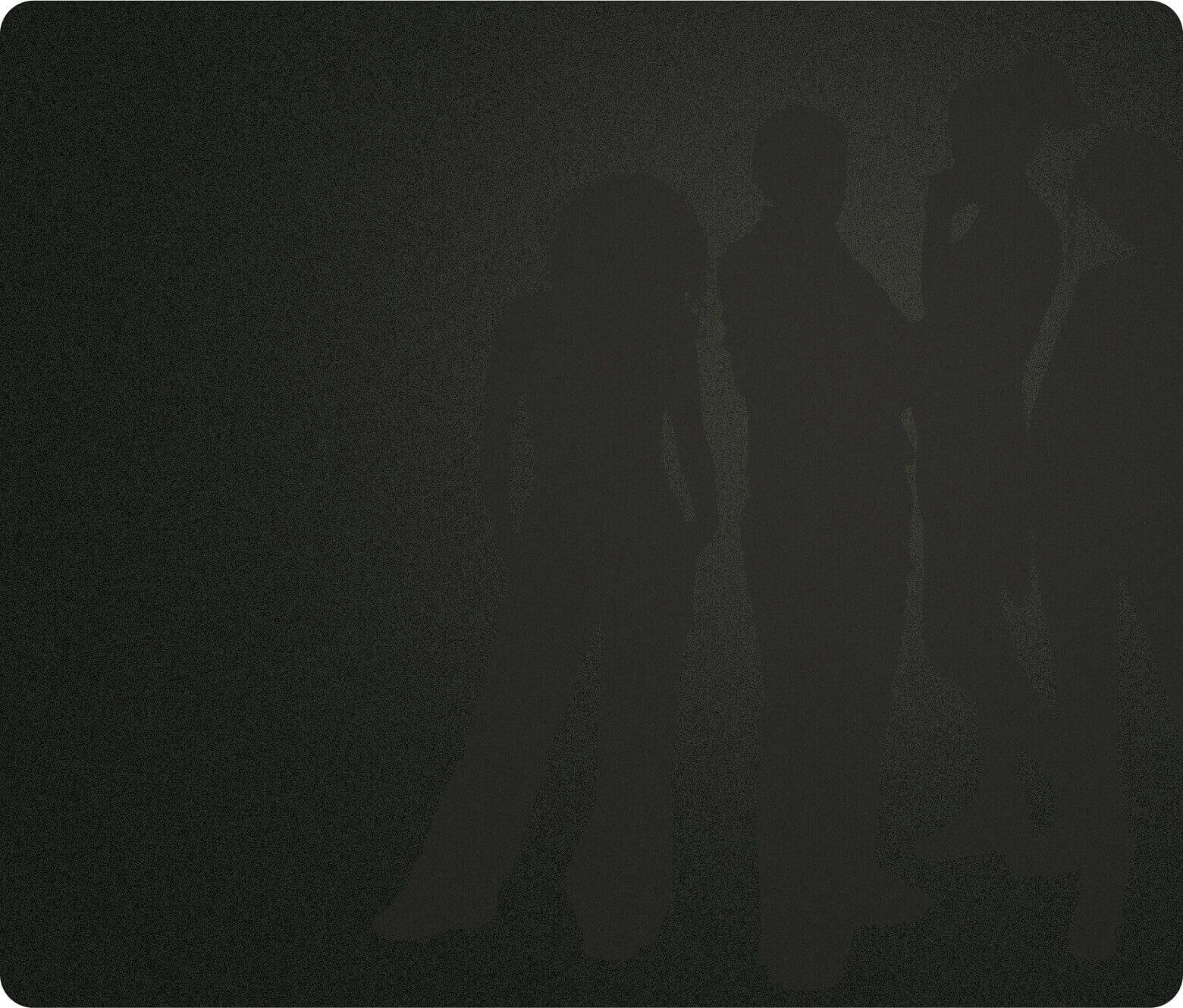

Thank you!
Read more articles
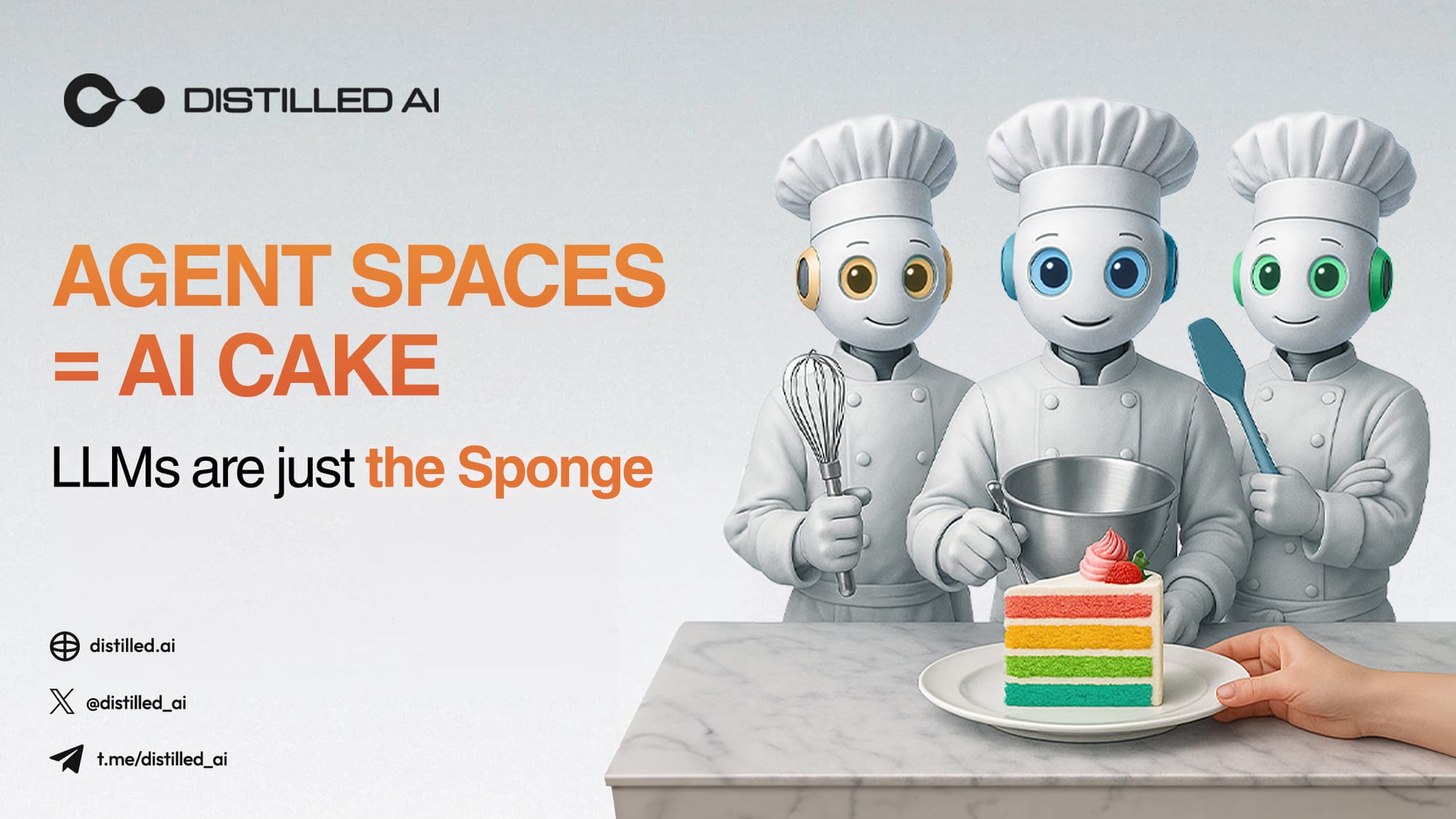
LLMs Are Just the Sponge: Building the Full AI Cake with Agent Spaces
From LLMs to AI systems is a long journey. Just like a sponge alone doesn't make a cake, LLMs alone can't create the rich, layered AI experiences we rely on today. This article explores how the AI Cake is formed—how modular systems, orchestrated workflows, and agent spaces bring everything together. And more importantly, what Distilled AI is aiming to build: the infrastructure that transforms these ingredients into a scalable, composable AI economy. The Secret Recipe When most people interact
MAY 14, 2025
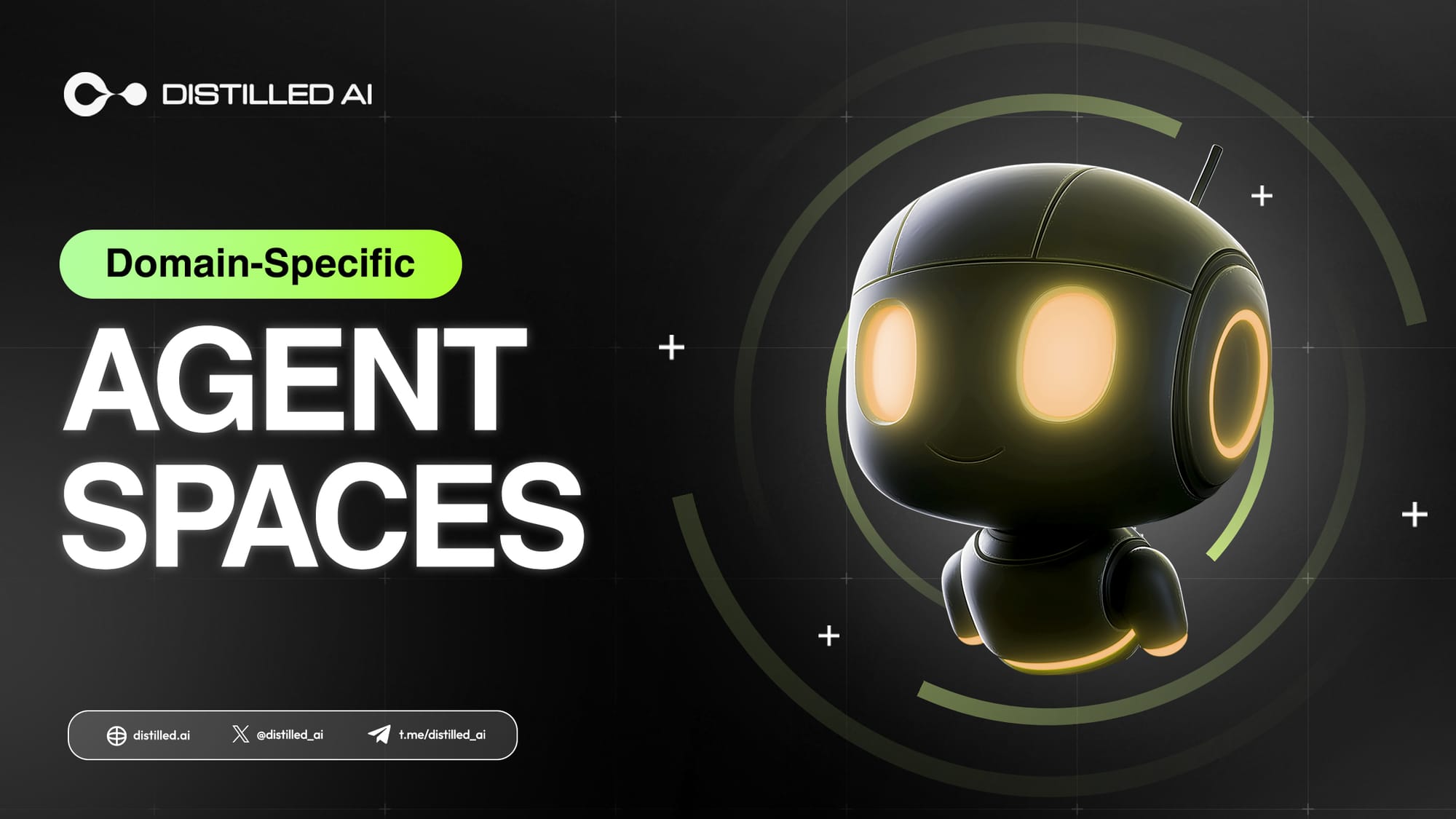
One Size Fits None: Why Domain-Specific Agent Spaces Win
As Agent Spaces begin to define how humans and AI agents collaborate, a critical design choice emerges: why not build a single Agent Space that does everything? A universal platform might sound efficient, but in practice, specialization beats generalization when solving hard problems. This article makes the case for domain-specific Agent Spaces—where modularity, focus, and community expertise create compounding value that no "do-everything" platform can match. Domain Specialization: The Compet
MAY 14, 2025